Debiasing Word Embeddings- Anchi Hsin
Presentation on “Man is to Computer Programmer as Woman is to Homemaker”?








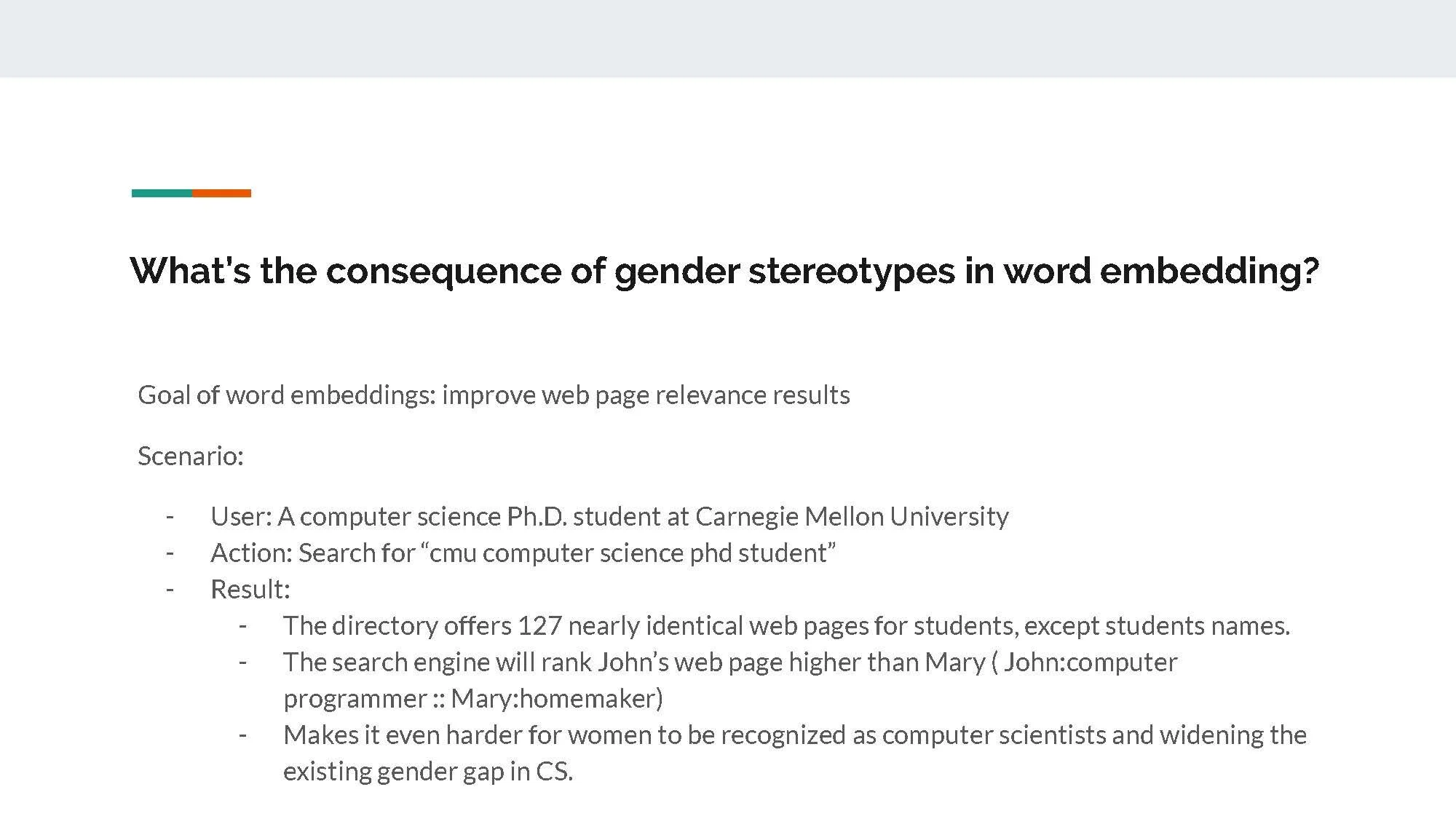












Notes on “Gender Shades: Intersectional Accuracy Disparities in Commercial Gender Classification”
Since the paper “Gender Shades: Intersectional Accuracy Disparities in Commercial Gender Classification” (presented by Nathalia Kasman) also referred and related to my readings (both have similar concept: making AI less bias and more inclusive), I pull out some of its interesting parts.
Darker-skinned females are the most misclassified group (with error rates of up to 34.7%). The maximum error rate for lighter-skinned males is 0.8%.
While face recognition software by itself should not be trained to determine the fate of an individual in the criminal justice system, it is very likely that such software is used to identify suspects.
The authors used Word2Vec to train an analogy generator that fills in missing words in analogies. The analogy man is to computer programmer as woman is to “X” was completed with “homemaker”, conforming to the stereotype that programming is associated with men and homemaking women.
A yearlong research investigation across 100 police departments revealed that African-American individuals are more likely to be stopped by law enforcement and be subjected to face recognition searches than individuals of other ethnicities
Advances gender classification benchmarking by introducing a new face dataset composed of 1270 unique individuals that is more phenotypically balanced on the basis of skin type than existing benchmarks.
The first intersectional demographic and phenotypic evaluation of face-based gender classification accuracy. Instead of evaluating accuracy by gender or skin type alone, accuracy is also examined on 4 intersectional subgroups: darker females, darker males, lighter females, and lighter males
Past research has also shown that the accuracies of face recognition systems used by US-based law enforcement are systematically lower for people labeled female, Black, or between the ages of 18—30 than for other demographic cohorts (Klare et al., 2012)
A binary ethnic categorization scheme: Caucasian and non-Caucasian (Farinella and Dugelay, 2012)
First, subjects’ phenotypic features can vary widely within a racial or ethnic category. For example, the skin types of individuals identifying as Black in the US can represent many hues. Thus, facial analysis benchmarks consisting of lighter-skinned Black individuals would not adequately represent darker-skinned ones. Second, racial and ethnic categories are not consistent across geographies: even within countries these categories change over time.
Since race and ethnic labels are unstable, we decided to use skin type as a more visually precise label to measure dataset diversity. Skin type is one phenotypic attribute that can be used to more objectively characterize datasets along with eye and nose shapes. Furthermore, skin type was chosen as a phenotypic factor of interest because default camera settings are calibrated to expose lighter-skinned individuals (Roth, 2009). Poorly exposed images that result from sensor optimizations for lighter-skinned subjects or poor illumination can prove challenging for automated facial analysis. By labeling faces with skin type, we can increase our understanding of performance on this important phenotypic attribute.
PPB is highly constrained since it is composed of official profile photos of parliamentarians. These profile photos are taken under conditions with cooperative subjects where pose is relatively fixed, illumination is constant, and expressions are neutral or smiling. Conversely, the images in the IJB-A and Audience benchmarks are unconstrained and subject pose, illumination, and expression by construction have more variation.
The six-point Fitzpatrick classification system which labels skin as Type I to Type VI is skewed towards lighter skin and has three categories that can be applied to people perceived as White
A board-certified surgical dermatologist provided the definitive labels for the Fitzpatrick skin type. Gender labels were determined based on the name of the parliamentarian, gendered title, prefixes such as Mr or Ms, and the appearance of the photo.
We intentionally choose countries with majority populations at opposite ends of the skin type scale to make the lighter/darker dichotomy more distinct.